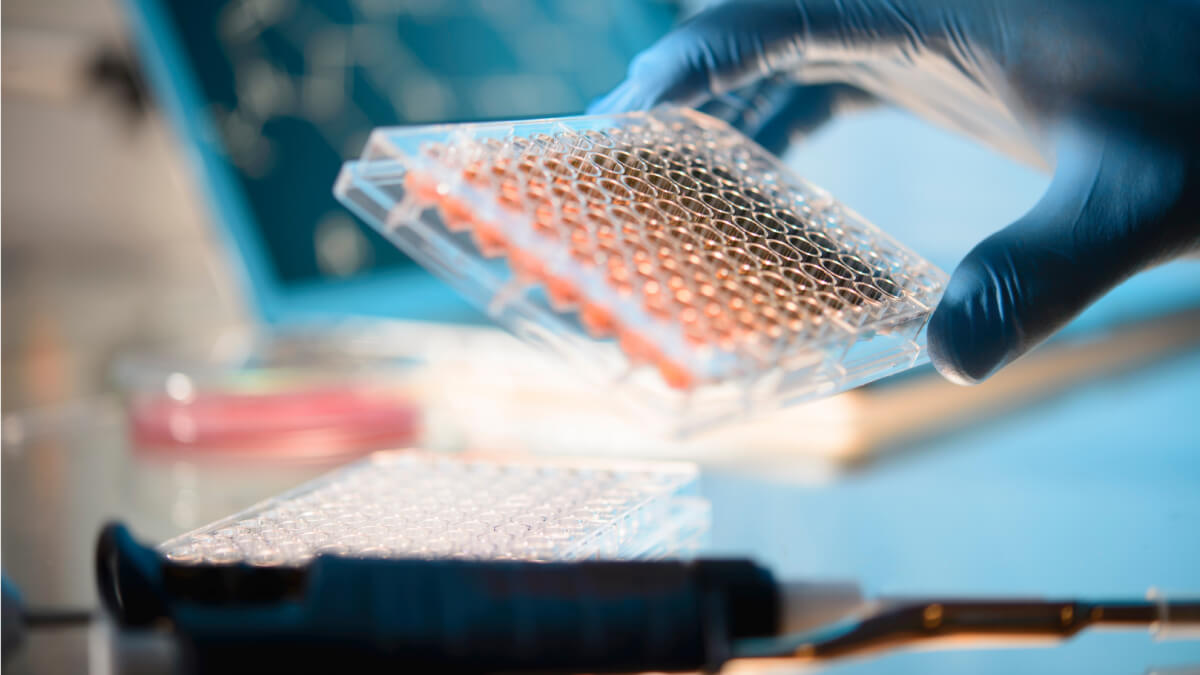
As a data scientist, whenever I built a machine learning model, I thought carefully about reproducibility. Where should I get the data from? Where can I store the proprietary data so that it's safe, but I'll be able to pull it again if I need to reproduce my results? How can I save the versions of the data analysis and machine learning packages so that a different person can fork my code and get the same results? How do I translate all of this data into something anyone can understand?
These, coincidentally, were also some of the fundamental questions that I used to ask when I worked in academic and industry labs that were developing next-generation materials for the clean energy industry.
The difference is that reproducibility, and thinking about reproducibility, are common in data science. But in science R&D, it's a rarity.
There is a frightening amount of irreproducible science being released into the world today. In fields like psychology where only 10% of research is reproducible. Even in cancer biology, only 40% is reproducible. Several years ago, Amgen reported that it could only replicate 6 out of 53 landmark cancer research papers.
This isn't only a problem with reproducing other people's work — in a Nature survey of 1,576 researchers, more than half of those surveyed have in the past failed to reproduce their own experiments.
There are many reasons for this, including:
- Structural issues
- Intense competition and career pressure to publish often leads scientists to make reproducibility a secondary priority.
- Lack of mandatory reproducibility guidelines to follow when publishing.
- Process issues
- Insufficient mentoring and oversight from colleagues and managers
- Poor peer-review process (reviewers aren't paid for their time and not the best researchers are reviewing manuscripts)
- Fundamental research issues
- Not enough effort put into replication before publishing (leading to low statistical power)
- Poor experimental design, leading to incorrect conclusions
- Lack of thorough reporting of methods, materials, equipment, and data, making the experimental process subject to undefined variables and human interpretation.
- Human errors due to carelessness, limitations of ability, or environmental factors
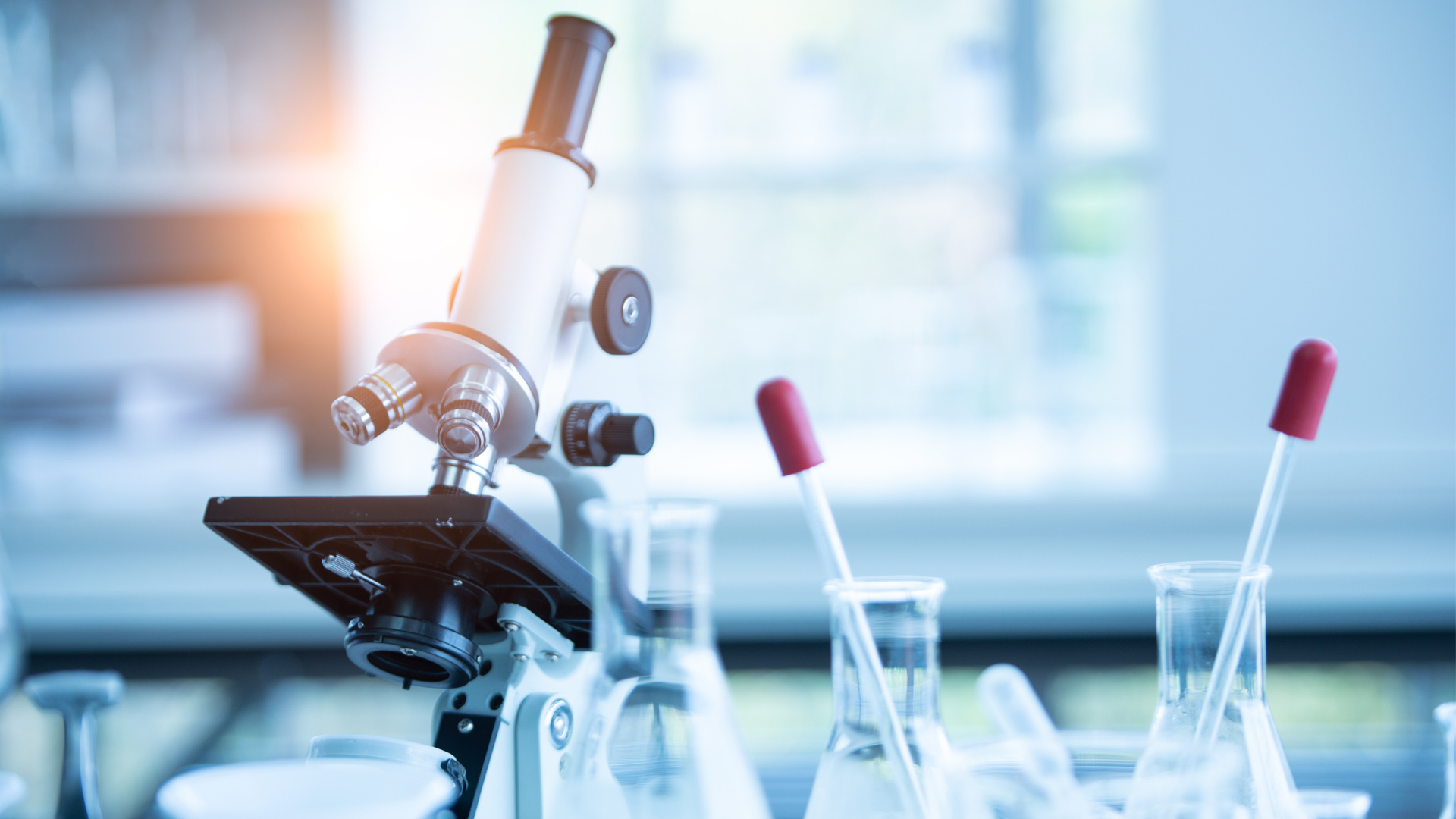
Bad science does have real-world implications. Take, for example, the Centers for Disease Control and Prevention's (CDC) stumbles in 2020 with COVID-19, with its flawed COVID-19 tests in February, and confusing guidance on aerosol transmission of coronavirus in September. The public trust in the CDC radically diminished, with the percentage of the public that holds "a great deal" of trust in the CDC dropping to 19% that September, down from 46% in March and 70% from before the pandemic. Or, take for example the previously mentioned Amgen study – dozens of these preclinical papers led to hundreds of secondary publications that built on the original work without first confirming the errors of the original paper. Much of this research led to clinical studies – which suggests that patients volunteered themselves for trials that likely wouldn’t work.
For any scientist and anyone who cares about the future of science, this is frightening. This is dangerous. People need to believe scientists. People who publish unreproducible research, whether or not it’s intentional, hurts the future of science.
The encouraging news is that there is a desire to do better science. According to the same Nature survey as above, one-third of respondents said that their labs have been taking concrete steps to improve reproducibility, including redoing work and asking someone else to reproduce the work within a lab. Scientists have also been beefing up their documentation and standardization of experimental methods.
Active collaboration between peers makes it more likely that errors are caught. It's the scientist's equivalent of the software engineer's Linus's Law: "With enough eyes, all software bugs become shallow." We see this with the growth of preprints by 142% in the last year, on sites like arXiv, MedRXiv, and BioRXiv, where unpublished research is reviewed and critiqued by the community. We also see this with ELNs like Colabra, which is helping scientists bring together all of their different data types and data formats to make collaboration with colleagues easier. Tools like Clustermarket that enables data collection with connected instruments are essential as well. Decades of tradition should not supersede changes that have been made attainable by recent innovations in software.
The larger challenge is that scientists themselves need to be aware of how to do better science. Tackling this challenge is the job of today's entrepreneurs and builders. We must be cognizant of building something designed for incentivizing scientific best practices while also being intuitive for researchers who may have done their science the same way for decades.